Semiconductors: Tailwinds from tectonic shifts in the AI compute sands
The increasing use of AI has positive implications for the semiconductor sector as the need for compute power and capital expenditure intensifies, reasons Portfolio Manager Richard Clode.
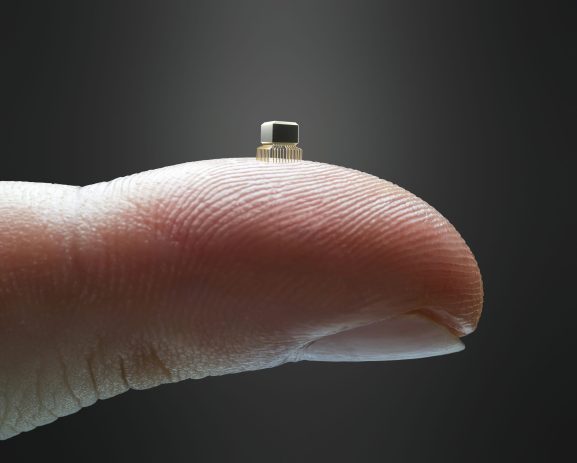
5 minute read
Key takeaways:
- The migration to the public cloud is aggregating compute for hyperscalers on a scale not witnessed before, and is becoming more cost effective and AI centric.
- AI performance requirements are driving increasing innovation in semiconductors to meet customers’ performance and power needs.
- The Global Technology Leaders Team believes the inflection in generative AI and the tectonic trends will create a wealth of broader investment opportunities over time.
The computer industry is going through two simultaneous transitions — accelerated computing and generative AI. A trillion dollars of installed global data center infrastructure will transition from general purpose to accelerated computing as companies race to apply generative AI into every product, service and business process. Jensen Huang, nVIDIA CEO
nVIDIA’s recent Q1 results and positive Q2 revenue guidance1 and subsequent stock reaction and positive news flow put the market-leading chip designer’s valuation in the rarefied air of $1 trillion market capitalisation. We think this is an appropriate time to reflect on the state of artificial intelligence (AI), its development and progress, as well as how to invest in companies that are benefiting from this critical juncture for technology. nVIDIA highlighted several salient themes that are noteworthy for active, long-term technology investors like us.
Migration to the public cloud is aggregating compute for hyperscalers on a scale not witnessed before
For decades, datacentres were the preserve of processors designed on the x86 architecture, dominated by Intel and more recently AMD. However, there are two major inflection points occurring. Firstly, the migration to the public cloud aggregates compute (computation and processing) at the hyperscalers on a scale not witnessed before. That scale, combined with the resources and technology acumen of those companies is leading them to move in two complementary directions as they focus on processing those cloud workloads more efficiently, notably around reducing power consumption, given this is one of the major costs for datacentres.
Source: Janus Henderson Investors, as at 31 May 2023. NVIDIA as at 31 May 2022. For illustrative purposes and not indicative of any actual investment.
Cloud acceleration leverages the parallel processing capabilities of graphics processing units (GPUs) or field programmable gate arrays (FPGAs) to offload compute from the central processing unit (CPU) to more power-efficient processors for the workload at hand. At the same time, hyperscalers are adopting Arm processors bringing their low-power processing, demonstrated for years in smartphones, to the datacentre via internally-designed custom semiconductors for example at Amazon, start-ups like Ampere, or the new Grace CPU launching later this year from nVIDIA.
The cloud as an enabler for AI
The second major inflection is the shift in compute workloads within the cloud to become AI centric. That is a very different workload to the traditional consumer internet workflow and consequently requires a different compute and datacentre design. So we are currently witnessing the hyperscalers speedily pivot their capital expenditure (capex) spending to the new AI age. AI training has aways utilised GPUs but generative (creation of new content) AI is also much more compute intensive on the inferencing side (running data points into an algorithm to calculate output) as well. A ChatGPT response to a query is much more computationally intensive than a Google keyword search. While Google has designed internal AI inferencing chips, to date a lot of AI inferencing had been done on x86 CPUs but that is not possible anymore for performance and cost reasons. That is also driving a shift to GPUs and custom silicon more tailored to this radically-different workload.
Implications for capital spending
The combination of the above is dramatically changing where the hyperscalers are spending their capex budgets. This is reflected by nVIDIA’s guidance for Q2 datacentre sales that were almost $4bn ahead of market expectations. To put this in perspective, Intel’s first quarter datacentre revenues were around $4bn and it made its first ever loss having lost market share and margins to AMD. Getting these tectonic shifts right can define investment returns.
Chip innovation is ramping up to provide better performance and power
As we look ahead we believe we are in the early stages of some significant wider semiconductor industry inflections. For years the exponential cost increase of maintaining Moore’s Law led to a rapidly shrinking pool of customers willing to pay up for bleeding edge (new and not fully tested) semiconductors. That trend is now reversing as AI performance requirements drive more customers to seek out the best performance and power. Taiwan Semiconductor Manufacturing Company (TSMC) has said it has twice the tape outs (chip designs ready for fabrication) on their current 3nm-class manufacturing process compared to the prior node. Many of those tape outs will be custom silicon designs from the hyperscalers. The complexities of creating such large, powerful chips is testing Moore’s Law to the limit, creating the ‘More than Moore’ trend and we are seeing incredible innovation here. The new MI300 from AMD launching later this year integrates multiple CPU and GPU chiplets, as well as high bandwidth memory via a new technology called hybrid bonding, pioneered by Besi.
Ultimately, as generative AI scales out, not all the compute can be done in centralised datacentres, therefore increasingly inference has to be done locally on edge devices, has lower latency (delays) and is better able to protect personal data. Qualcomm is currently demonstrating the ability to inference Meta’s LLaMA large language model on a smartphone. nVIDIA has also demonstrated that the complexity of AI requires a full stack (complete) solution so the innovation will not just be in hardware but also in software, for example its new Hopper chips have a transformer software engine that intelligently balances the trade-off between computational precision and accuracy to maximise the speed AI models can be trained.
Summing it all up, we believe the next major compute wave is upon us with the inflection in generative AI and the tectonic trends outlined above will create a wealth of broader investment opportunities over time across a number of companies that are well positioned to benefit.
1 nVIDIA financial results first quarter fiscal year 2024, announced 24 May 2023.
AI inference: the first phase of machine learning is the training phase where intelligence is developed by recording, storing, and labeling information. In the second phase, the inference engine applies logical rules to the knowledge base to evaluate and analyse new information, which can be used to augment human decision making. Compute: relates to processing power, memory, networking, storage, and other resources required for the computational success of any programme. CPU: the central processing unit is the control center that runs the machine’s operating system and apps by interpreting, processing and executing instructions from hardware and software programmes. Edge device: a network component responsible for connecting the local area network to an external and wide area network. FGPA: Field Programmable Gate Arrays are integrated circuits often sold off-the-shelf that provide customers the ability to reconfigure the hardware to meet specific use case requirements after the manufacturing process, including upgrades and bug fixes. GPU: a graphics processing unit performs complex mathematical and geometric calculations that are necessary for graphics rendering. Hyperscalers: companies that provide infrastructure for cloud, networking, and internet services at scale. Examples include Google Cloud, Microsoft Azure, Meta Platforms, Alibaba Cloud, and Amazon Web Services (AWS). Moore’s Law: coined in 1965 by Intel co-founder Gordon E. Moore, it is the ability to roughly double the number of transistors that can fit onto a chip (aka integrated circuit), enabling technology to become smaller, faster, and cheaper over time. More than Moore: instead of “more Moore” (further miniaturisation), “more than Moore” addresses the physical limitations of Moore’s Law by combining digital and non-digital functions on the same chip. Workload: the amount of processing that a computer has been given to do at a given time.
IMPORTANT INFORMATION
Technology industries can be significantly affected by obsolescence of existing technology, short product cycles, falling prices and profits, competition from new market entrants, and general economic conditions. A concentrated investment in a single industry could be more volatile than the performance of less concentrated investments and the market.
These are the views of the author at the time of publication and may differ from the views of other individuals/teams at Janus Henderson Investors. References made to individual securities do not constitute a recommendation to buy, sell or hold any security, investment strategy or market sector, and should not be assumed to be profitable. Janus Henderson Investors, its affiliated advisor, or its employees, may have a position in the securities mentioned.
Past performance does not predict future returns. The value of an investment and the income from it can fall as well as rise and you may not get back the amount originally invested.
The information in this article does not qualify as an investment recommendation.
Marketing Communication.